-
大小: 8.55MB文件类型: .zip金币: 2下载: 0 次发布日期: 2023-10-14
- 语言: 其他
- 标签: DeepLearning
资源简介
cnn_utils.py、test_signs.h5、train_signs.h5,亲测!
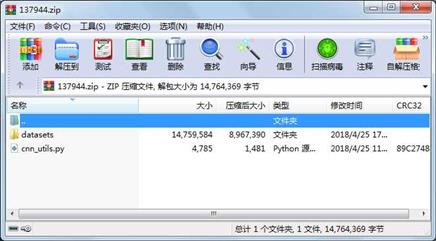
代码片段和文件信息
import math
import numpy as np
import h5py
import matplotlib.pyplot as plt
import tensorflow as tf
from tensorflow.python.framework import ops
def load_dataset():
train_dataset = h5py.File(‘datasets/train_signs.h5‘ “r“)
train_set_x_orig = np.array(train_dataset[“train_set_x“][:]) # your train set features
train_set_y_orig = np.array(train_dataset[“train_set_y“][:]) # your train set labels
test_dataset = h5py.File(‘datasets/test_signs.h5‘ “r“)
test_set_x_orig = np.array(test_dataset[“test_set_x“][:]) # your test set features
test_set_y_orig = np.array(test_dataset[“test_set_y“][:]) # your test set labels
classes = np.array(test_dataset[“list_classes“][:]) # the list of classes
train_set_y_orig = train_set_y_orig.reshape((1 train_set_y_orig.shape[0]))
test_set_y_orig = test_set_y_orig.reshape((1 test_set_y_orig.shape[0]))
return train_set_x_orig train_set_y_orig test_set_x_orig test_set_y_orig classes
def random_mini_batches(X Y mini_batch_size = 64 seed = 0):
“““
Creates a list of random minibatches from (X Y)
Arguments:
X -- input data of shape (input size number of examples) (m Hi Wi Ci)
Y -- true “label“ vector (containing 0 if cat 1 if non-cat) of shape (1 number of examples) (m n_y)
mini_batch_size - size of the mini-batches integer
seed -- this is only for the purpose of grading so that you‘re “random minibatches are the same as ours.
Returns:
mini_batches -- list of synchronous (mini_batch_X mini_batch_Y)
“““
m = X.shape[0] # number of training examples
mini_batches = []
np.random.seed(seed)
# Step 1: Shuffle (X Y)
permutation = list(np.random.permutation(m))
shuffled_X = X[permutation:::]
shuffled_Y = Y[permutation:]
# Step 2: Partition (shuffled_X shuffled_Y). Minus the end case.
num_complete_minibatches = math.floor(m/mini_batch_size) # number of mini batches of size mini_batch_size in your partitionning
for k in range(0 num_complete_minibatches):
mini_batch_X = shuffled_X[k * mini_batch_size : k * mini_batch_size + mini_batch_size:::]
mini_batch_Y = shuffled_Y[k * mini_batch_size : k * mini_batch_size + mini_batch_size:]
mini_batch = (mini_batch_X mini_batch_Y)
mini_batches.append(mini_batch)
# Handling the end case (last mini-batch < mini_batch_size)
if m % mini_batch_size != 0:
mini_batch_X = shuffled_X[num_complete_minibatches * mini_batch_size : m:::]
mini_batch_Y = shuffled_Y[num_complete_minibatches * mini_batch_size : m:]
mini_batch = (mini_batch_X mini_batch_Y)
mini_batches.append(mini_batch)
return mini_batches
def convert_to_one_hot(Y C):
Y = np.eye(C)[Y.reshape(-1)].T
return Y
def forward_propagation_for_predict(X parameters):
“““
Implements the forward propagation for the model: LINEAR -> RELU -> LINEA
属性 大小 日期 时间 名称
----------- --------- ---------- ----- ----
目录 0 2018-04-25 17:32 datasets\
文件 1477712 2018-04-16 15:39 datasets\test_signs.h5
文件 13281872 2018-04-16 15:38 datasets\train_signs.h5
文件 4785 2018-04-25 11:07 cnn_utils.py
- 上一篇:吴恩达老师深度学习第二课第三周2-3资源文件
- 下一篇:车载双向DCDC
相关资源
- 机器学习个人笔记完整版v5.2-A4打印版
- Neural Networks and DeepLearning - Michael Nie
- PyTorch介绍及入门pdf
- NeuralNetworksDeepLearning_jb51.rar
- 基于深度学习人脸识别
- 吴恩达老师深度学习第二课第三周2
- 1825705zw_DeepLearning深度学习学习笔记整
- 吴恩达神经网络和深度学习,第一课
- Deep Learning For Dummies
- 斯坦福大学-深度学习-cs230-DeepLearnin
- 吴恩达深度学习deeplearning第一课课后
- 深度学习DeepLearning中文+英文版
- 吴恩达 Deeplearning深度学习笔记v5.41.
- deep learning中文版花书
- deeplearning深度学习中文版无水印
- DeepLearningwithTensorFlow2nd+DeepLearningwith
- 《DeepLearning》深度学习圣经-IanGoodfe
- 《DeepLearning》深度学习圣经-IanGoodfe
- Deep-Learning-For-Computer-Vision-第一册sta
- 深度学习500问PDF.zip
- 包含了轮船,游船,渔船,帆船的普
- 吴恩达deeplearning.ai项目前两课程的全
- caffe imagenet_mean.binaryproto
- 吴恩达深度学习deeplearning第五课第一
- DeepLearninginNaturalLanguageProcessing.pdf
- Deeplearning(中文版)165626
- 深度学习DeepLearning
- Deeplearning4j官方手册
- TensorFlow For Machine Intelligence(非扫描版
- 动手学深度学习源代码
评论
共有 条评论